Columns
Word from Wall Street: New Forecasting Tools
Internet search data have been accurate in forecasting sales of specific supplement product categories. But common sense still needs to be applied in analysis.
By: Adam Ismail
Executive Director, Global Organization for EPA and DHA Omega-3s (GOED)
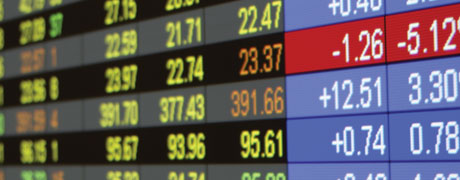
At the end of the day, valuations are based on expectations of the future, whether it is in the stock markets or in mergers and acquisitions. There are dozens of economic indicators that analysts use to predict the future, such as GDP, consumer confidence, retail sales indices, etc. If you are interested in learning more about what all of these mean, I highly recommend buying “The Atlas of Economic Indicators” by Carnes and Slifer.
However, these indicators generally focus on either the larger economy or major sectors of the economy (e.g., manufacturing and retail sales), and do not help you forecast how nutraceuticals sales are doing. Additionally, these macro-indicators generally look backward in time, are released many months after the time period they are reporting on, and the ones that do look forward are based on opinion polls rather than actual data.
Of course there are data sources you can buy to tell you how nutraceuticals are doing, such as retail scan data, but even that has its limitations because there is still a 1-2 month lag in reporting times and it focuses on single channels. So how do you monitor consumer interest in real time? In the wonderful information age, there is, theoretically, more information that can be used to measure this. Google has postulated that data from Internet searches can be used as indicators of interest (www.google.com/finance/domestic_ trends). In fact, Google has even attempted to use search data to predict how the economic indicators will look, before they are released. So can Internet search data be used to forecast and monitor nutraceutical sales? Logic says that if consumers are worried about a health condition, then they will search out information on products, including dietary supplements, that can help. So if Internet searches spike, it could correlate with increased sales.
Google is so dominant in Internet searches that its data provides a good look into what consumers are searching for. It tracks and reports search data on a weekly basis going back to 2004. The most comprehensive source of data on various supplements is Nutrition Business Journal, but NBJ data on supplement sales are reported on an annual basis, so it does not line up perfectly. However, we can take the weekly Google Insights data (www.google.com/insights/search), create an average value for each year, and then see if it correlates with NBJ data.
What we find is that Google’s information is a better indicator for certain supplements compared to others, but when it is accurate it has been extremely accurate. To determine accuracy you want to do a regression comparing the Google search index for a particular search term, in our case a supplement name, and the NBJ sales data for that supplement. The R2 statistic is a measurement of how well a regression run on two or more variables explains the results. Strong regressions will typically have an R2 greater than 0.70. Put another way, a strong regression equation looking at the Google search indices for a supplement will be able to explain at least 70% of the annual NBJ sales numbers for a supplement. If it cannot explain more than that, then it generally means either that single variable would not be a good proxy for estimating sales, or that more variables are needed to explain them.
Table 1 shows the R2 statistics for a number of supplements. In addition, we have used the year-to-date Google Search index numbers to predict the approximate sales for 2009. When NBJ releases its 2009 numbers, we will revisit this and see how accurate it was, but we have seen scan data that indicates it is generally accurate.
So why can you get a strong correlation with some products and not others? There are three examples in the table to look at: noni, ginkgo and garlic. Noni has a low R2 statistic, which means that the forecasted growth for 2009 using the year-to-date search data is not likely to be accurate. Most noni though is sold in the network marketing channel, which is the least visible of all channels, and the market for these products is highly concentrated. This means that it may be more difficult to estimate how large the market really is because companies are less likely to share data. Network marketers also depend heavily on international markets, so statistics on the overall growth of the market may not necessarily apply in the U.S., which is what NBJ data try to estimate and is from where the searches we used originated. In addition, products that are primarily sold in the network marketing channel are typically sold person-to-person. So Internet search data may not be the best proxy for this type of sale.
Ginkgo is another interesting case. In this instance, we first looked at the search term“ginkgo biloba,”but found that ginkgo is actually a difficult word for consumers to spell, and in fact there were seven different misspelled permutations of “ginkgo biloba” that ranked higher in Google search statistics than the actual term. So in this case, you would have to experiment with various search strings that combine multiple terms to approximate the universe of searches.
Lastly, let’s look at garlic. Internet search data for garlic actually had a strong correlation with supplement sales, but you cannot always rely on numbers. A good dose of common sense is always important with statistics, and in this case you would quickly discover that there are a lot of cooking-related searches on garlic that are skewing the analysis. In fact, the Google Insights tool also shows you the top 10 similar searches, and all of them are food-related. So in the case of garlic, again more work would be needed before you could use this as a forecasting tool.
To summarize, Internet search terms have been extremely accurate in forecasting sales of specific supplement product categories, and regressions on these data appear to forecast sales accurately for products in the period before actual market estimates are released. However, there are limitations, and common sense needs to be applied to the analysis. Search terms may not be good proxies for products that are not well distributed across channels, nor would a single search term be a good proxy for products that could cross outside the realm of the supplement industry or could confuse consumers. In these cases more work needs to be done to pool a series of search terms to create an aggregate index that would represent consumer interest—and even then you may find nothing. However, in the case of products like fish oils or vitamin D, you would see the rapid growth of these products coming months in advance of actual data released. It will be interesting to see if this tool can be used to predict brand or company sales as well, which could of course be very useful in investing.